Part II: Overview
In the previous article, we discussed that AI has great potential to revolutionise the healthcare sector by deriving brand new insights from the data pool. Patients require protection from the elimination of bias built into algorithms, defective diagnosis, and unacceptable use of personal data. However, healthcare AI regulation is still in its infancy stage, and regulators are catching up to it. There is still no concrete law in this area; The US and the EU have taken tentative steps highlighting the need for regulation and issuing proposals. The main reason behind this is the difficulty in regulating dynamic technology. In this article, we will investigate the standardised sets of Regulations for AI/ML in healthcare and life science industries that different actions bodies have set up serially.
(i) European Commission: Artificial Intelligence Act
European Commission issued a proposal for a Regulation of the European Parliament on artificial intelligence (EU Artificial Intelligence Act) on 21st April 2021 [R1]. The proposal focuses on the various important aspects such as Harmonised rules for the market and applying them to services and the use of AI systems in the EU. Another important topic discussed is the prohibition of certain AI practices, specific requirements for high-risk AI systems and the rules for operators of such systems, harmonised transparency rules for AI systems which are used for interactions with natural persons, emotion recognition systems and biometric categorisation systems, etc.
In addition, Annex I [R2] of the proposal lists the different techniques and approaches used to develop an AI model. It includes:
- Machine Learning – Supervised, unsupervised and reinforcement learning.
- Logic & Knowledge-based Approaches – Knowledge representation, inductive (logic) programming, knowledge bases, inference, deductive engines, (symbolic) reasoning and expert systems.
- Statistical Approaches – Bayesian estimation, search, and optimisation methods.
These techniques and approaches are part of the definition of AI formulated by the European Commission. This definition includes if an AI (Artificial Intelligence) system as software is developed with one or many approaches listed in Annex I and if it can generate outputs for a given human-defined objective.
Furthermore, in the EU Artificial Intelligence Act, AI is assessed according to a Risk-Based Approach which aims to categorise four types of risks affecting fundamental rights and users’ safety, namely:
- Unacceptable risk (Title II, Article 5): Prohibited AI practices, which include:
-
- Systems which deploy subliminal techniques.
- Systems that exploit specific vulnerable groups (physical or mental disability).
- Systems used by public authorities, or on their behalf, for social scoring purposes.
- ‘Real-time’ remote biometric identification systems used in publicly accessible spaces.
- High risk (Title III, Article 6): High-risk AI systems impact people’s safety or fundamental rights. The draft distributes into two categories:
-
- High-risk AI systems are used as a safety component of a product or as a product falling under Union health and safety harmonisation legislation.
- High-risk AI systems deployed in eight specific areas identified in Annex III [R2].
- Limited risk (Title IV) – the systems presenting limited risk, such as human interactive systems, emotion recognition systems, biometric categorisation systems, etc.
- Low or Minimal risk (Title V)– Any other systems with low or minimal risk could be developed without any additional legal obligation.
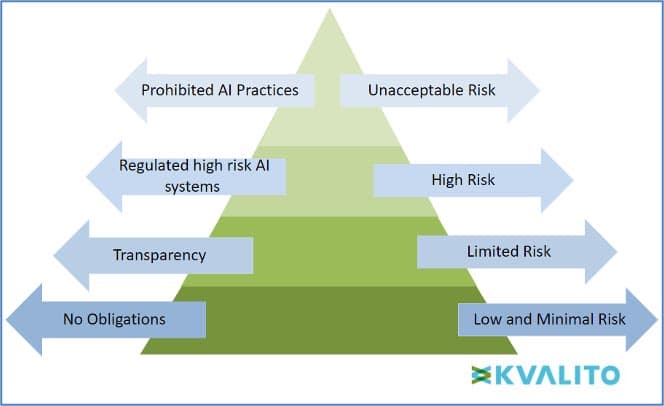
Figure 1: The EU’s draft AI regulations classify AI systems into four risk categories.
theThe systems with high risk are of most concern and should be regularised in the EU with a standard, harmonised set of rules in the industry. The proposal highlighted the rules as follows.
The set of new rules which the high-risk AI systems need to obey includes:
- Registration of the high-risk systems in an EU-wide Database managed by the Commission before placing them into service (in the case of medical devices).
- Conformity assessment on their own by EU legislation for the verification of compliance with the new requirements. CE marking is used for this purpose.
- Compliance with a range of requirements, particularly on risk management, testing, technical robustness, data training and data governance, transparency, human oversight, and cybersecurity with a range of requirements particularly on risk management, testing, technical robustness, data training and data governance, transparency, human oversight, and cybersecurity (mentioned on Articles 8 to 15). An authorised representative will be appointed for this purpose.
Other than this, all the EU harmonised legislations related to risk-based AI systems are listed in Annex II, including Regulation (EU) 2016/425 [R3] on personal protective equipment, Regulation (EU) 2017/745 [R4] on medical devices, Regulation (EU) 2017/746 [R5] on in vitro diagnostic medical devices and many others.
(ii) FDA Discussion Paper
(a) Proposed Regulatory Framework for Modifications to Artificial Intelligence/ Machine Learning-Based Software as a Medical Device (SaMD)
Other than the European Commission, the US Food and Drug Administration (FDA) published “Proposed Regulatory Framework for Modifications to Artificial Intelligence/Machine Learning (AI/ML)-Based Software as a Medical Device (SaMD) – Discussion Paper and Request for Feedback.” [R6] in 2019 proposed some modifications in the AI/ML system as a SaMD and approach to premarket review for AI and ML-driven software modifications.
According to the 510(k) Software Modification Guidance [R7] in the discussion paper, the risks to users from the software changes can be identified. Some categories of software modifications require a premarket submission; these types of changes are:
- A change that introduces a new risk or modifies an existing risk could result in significant harm.
- A change to risk controls to prevent significant harm
- A change that significantly affects clinical functionality or performance specifications of the device.
In addition, the discussion paper points out that, after many modifications, AI/ML-based SaMD needs retraining of the algorithms with new datasets. According to the software modification guidance, these would be subjected to premarket review.
There are three types of AI/ML Based Modifications listed in the discussion paper by the FDA:
- Modifications related to PERFORMANCE, with no change to the intended use or new input type of the SaMD: When a modification is performed for only improvements to analytical and clinical performance. This type of change may demand retraining the system with new datasets, change in the model architecture, etc. (e.g., increased sensitivity of the SaMD at detecting breast lesions suspicious for cancer in digital mammograms).
- Modifications related to INPUTS, with no change to the intended use: The type of modifications that changes the inputs used by the AI/ML system. For Example: Expanding the SaMD’s compatibility with other sources of the same input data type (e.g., SaMD modification to support compatibility with CT scanners from additional manufacturers).
- Modifications related to the SaMD’s INTENDED USE: These types of modifications include those that result in a change in the significance of the information provided by the SaMD (e.g., from a confidence score that is ‘an aid in diagnosis’ (drive clinical management) to a ‘definitive diagnosis’ (diagnose)).
Hence from these understandings, the proposed discussion paper containing the different modification types helps develop an AI/ML-based SaMD.
(b) Artificial Intelligence/ Machine Learning-Based Software as a Medical Device Action Plan
Based on the proposed framework in the FDA discussion paper from 2018, along with stakeholder response and recommendations from International Medical Device Regulators Forum (IMDRF) [R8], an action plan was set up and published by FDA in January 2021, “Artificial Intelligence/Machine Learning (AI/ML)-Based Software as a Medical Device (SaMD) Action Plan.” [R9]. This outlines a holistic approach based on total product lifecycle oversight to further the enormous potential of the technology to improve patient care meanwhile delivering safe and effective software functionality. The regulations might evolve over time to stay current, address patient safety, and improve access to these promising technologies.
From the knowledge & understanding of the AI/ML discussion paper and application of those in a practical, real-world field FDA identifies the following actions:
- Tailored Regulatory Framework for AI/ML-based SaMD: Requirement for development of updates in the proposed regulatory framework as suggested in the AI/ML discussion paper and the issuance of a Draft Guidance on the Predetermined Change Control Plan.
- Good Machine Learning Practice (GMLP): Encouragement of harmonised development of GMLP through the participation of FDA in collaborative communities and consensus standards development efforts.
- Patient-Centred Approach Incorporating Transparency to Users: Supporting a patient-centred approach by continuing to host discussions on the role of transparency to users of AI/ML-based devices. Also, building the Patient Engagement Advisory Committee (PEAC), investigating patient trust in AI/ML systems, and organising public workshops.
- Regulatory Science Methods Related to Algorithm Bias & Robustness: To support regulatory science efforts on developing methodologies for improving and evaluating ML algorithms. This includes the steps to identify and eliminate bias (if any), and checking the robustness of these algorithms to deal with changing clinical inputs and conditions.
- Real-World Performance (RWP): Advancement of real-world Performance Pilots in association with FDA’s other programs and stakeholders to get more clarity on how a practical, real-world AI/ML-based SaMD can be.
These are the actions from the current Action Plan of the FDA, but there will be continuing evolution of the actions due to the incredible speed of progress in this field.
One of the actions (Action 2) deals with the harmonisation of Good Machine Learning Practice (GMLP) development which was then unveiled in October 2021 as “10 Guiding Principles” by FDA, MHRA and Health Canada on the circle of IMDRF (International Medical Device Regulators Forum) [R10] to drive the adoption of GMLP to promote safe, effective, and high-quality AI/ML medical devices. These principles lay the foundation for GMLP to address AI’s unique nature and help cultivate future growth in this rapidly progressing field.
(c) Good Machine Learning Practices for Medical Device Development: Guiding Principles
- Multi-Disciplinary Expertise Is Leveraged Throughout the Total Product Life Cycle.
This entails an in-depth understanding of how an AI/ML model’s intended integration into clinical workflow, as well as of benefits and risks to patients over the full course of the device’s lifecycle.
- Good Software Engineering and Security Practices Are Implemented.
Model design must adhere to good software engineering practices, data quality assurance, data management, and robust cybersecurity practices. These practices also cover risk, data management and design processes that are robust and clearly communicate design, implementation, and risk management rationales to ensure the integrity and authenticity of data.
- Clinical Study Participants and Data Sets Are Representative of the Intended Patient Population.
Data collection protocols should be designed to capture interesting patient population characteristics, and the results could be generalised across the intended patient population.
- Training Data Sets Are Independent of Test Sets.
Training datasets should be kept from test datasets separately to assure independence.
- Selected Reference Datasets Are Based Upon Best Available Methods.
This effort is to ensure the collection of clinically relevant data and understand the limitation of any reference data for promoting model robustness and generalizability.
- Model Design Is Tailored to the Available Data and Reflects the Intended Use of the Device.
Performance goals for testing should be based on well-understood clinical benefits and risks to assure the product is safe and effective.
- Focus Is Placed on the Performance of the Human-AI Team.
Other than model performance, human factors and the model output’s human interpretability should be considered.
- Testing Demonstrates Device Performance during Clinically Relevant Conditions.
While developing and executing test plans, factors such as intended patient populations, human-AI team interactions, measurement inputs and potential confounding factors need to be considered to generate clinically relevant device performance.
- Users Are Provided Clear, Essential Information.
AI/ML device developers and manufacturers are responsible for providing relevant, clear, and accessible information to intended users. The data includes instructions for use, the performance of the AI/ML model for appropriate subgroups, model training and testing data characteristics and limitations. Device updates based on real-world performance monitoring should also be made available to users.
- Deployed Models Are Monitored for Performance, and Re-training Risks are Managed
This should be done to improve safety and performance, accompanied by periodic or continuous model training after deployment for more effective risk and safety management.
Conclusion:
In this article, we have explored different aspects of AI/ML in the pharma and healthcare industry. It is beyond any doubt that there are a considerable number of benefits of using AI/ML-based systems in this industry, and its application is already impacting the industry heavily. Still, every coin has two faces, and it is equally important that an individual be alert to the negative aspects of this growing technology. So it is highly recommended that AI/ML systems are effectively regulated before widespread market application.
Although the application of AI/ML can produce considerable transformations in the healthcare sector, the main challenge lies in navigating through the high volume of diverse data together whilst adhering to the regulatory requirements of the healthcare industry.
We have seen in detail what the different regulatory bodies have proposed and can conclude that by European Commission recommendations and mandates were given for the stakeholders and operators to keep a keen eye on the regulation fulfilment for the high-risk AI systems with more importance than a limited-risk or low-risk system, verification of compliance of systems using CE markings and/or authorised representatives, Registration of the systems in EU-wide Database, etc. FDA’s action plan focuses on the transparency of the systems to the customers, well-knit and oriented systems meeting specification compliances, and describes (in detail) the various guidelines about modifications adopted, GMLP, RWP, etc.
We will discuss the impact of these on the CSV/CSA world in the following article, ‘Artificial Intelligence Regulation and its impact on CSV/CSA (III)’.
References:
[R1] European Commission Artificial Intelligence Act: https://www.europarl.europa.eu/RegData/etudes/BRIE/2021/698792/EPRS_BRI(2021)698792_EN.pdf
[R2] Annexes to the European Commission Artificial Intelligence Act: https://artificialintelligenceact.eu/annexes/
[R3] Regulation (EU) 2016/425 https://eur-lex.europa.eu/legal-content/EN/TXT/PDF/?uri=CELEX:32016R0425&rid=4
[R4] Regulation (EU) 2017/745 https://eur-lex.europa.eu/legal-content/EN/TXT/?uri=CELEX%3A32017R0745
[R5] Regulation (EU) 2017/746 [R6] https://eur-lex.europa.eu/eli/reg/2017/746/oj
[R7] FDA Deciding When to Submit a 510(k) for a Software Change to an Existing Device
[R8] FDA International Medical Device Regulators Forum (IMDRF)
[R10] Good Machine Learning Practice for Medical Device Development: Guiding Principles https://www.fda.gov/media/153486/download
Authors: Aritra Chackrabarti, Dr. Anna Lena Hürter, Karunakar Budema, Jocelyn Chee
KVALITO is a strategic partner and global quality and compliance service and network for regulated industries. To find out more, please visit us at www.kvalito.ch . If you would like to benefit from KVALITO’s expert services, please send us an email at contact@kvalito.ch. Are you looking for an exciting and challenging position as a consultant, or maybe you are an ambitious student/graduate looking for an internship? Please send your complete application to recruiting@kvalito.ch.
Read the other articles of the series:
Taking Shape: Artificial Intelligence Regulation and its impact on CSV/CSA (I)
Taking Shape: Artificial Intelligence Regulation and its impact on CSV/CSA (III)